Accurate forecasting can have a major impact on various aspects of business, from resource and inventory management to sales, marketing, budgeting, and everything in between. It can help stakeholders make more informed decisions to drive their business forward, allowing it to stay competitive in the global marketplace.
Join us as we delve deep into what forecast accuracy is, why it is important, and what benefits it can bring to your business. And, please stay with us as we discuss different forecast accuracy formulas and how implementing them can help you make more accurate projections.
What Is Forecast Accuracy?
Forecast accuracy is a way of calculating the accuracy of a particular forecast (e.g., sales forecast) or how well it managed to predict real outcomes (e.g., sales values). It can also help companies assess how reliable and effective their current forecasting models, methods, and techniques are.
Calculating the degree to which a forecast is dependable can provide businesses with valuable insight into their current market (overall market and the most recent trends). This info can help companies (i.e., relevant stakeholders) in their decision-making process, making it more reliable and data-driven.
Forecast accuracy is in use across numerous industries and business fields, with the most common types of forecasting being:
- Sales forecasting — It helps companies predict their future sales volumes, allows them to enhance inventory management, and better organize production processes.
- Demand planning— Allows businesses to understand their inventory needs. The goal is to ensure product availability to meet customer demand without running out of inventory or having too much of it.
- Financial forecasting — Businesses use this type of forecasting to make decisions about budgeting, resource management, and allocation, various investment plans, and policies.
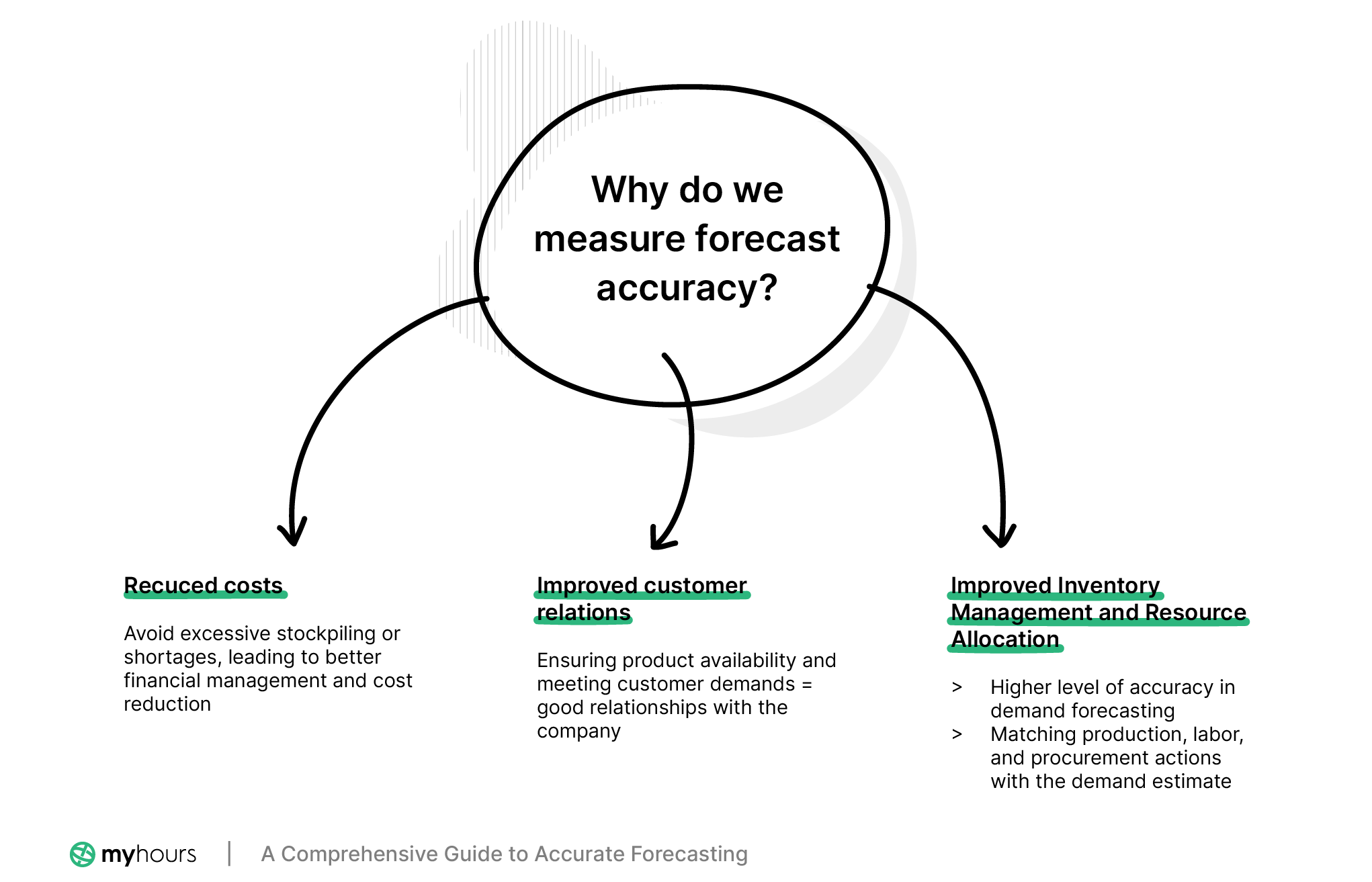
Why Is Forecast Accuracy Important for Business?
Comparing and contrasting the estimated values of a particular forecast with real-life outcomes gives insight into the quality of the forecasts, which is what forecast accuracy is all about. It allows businesses to measure how accurate their current forecasting models are and gives them enough information to discern how much confidence they should place in each forecasting model.
By evaluating and improving forecast accuracy regularly, companies can upgrade their planning processes and make better strategic decisions, allowing them to:
Reduce Costs
Forecast accuracy allows businesses to better manage their inventories and avoid excessive stockpiling or shortages, leading to better financial management and cost reduction. Once companies know their forecasting methods are reliable (through forecast accuracy), they can match their production capacity and procurement needs with a more dependable demand estimate.
This can help minimize holding and carrying costs and products becoming out-of-date (i.e., obsolescence), reducing overall expenses.
Improve Customer Relations
Forecast accuracy plays a pivotal role in ensuring product availability and meeting customer demands, which inevitably leads to better resource and supply chain management. When customers are certain they’ll receive their products on time and without hassle or delays, their overall relationships and experience with the company will improve.
Improve Inventory Management and Resource Allocation
Forecast accuracy directly impacts inventory management by allowing companies to determine the accuracy of demand forecasting (for a specific product or service). It’s also extremely helpful in resource allocation, allowing businesses to match production, labor, and procurement actions with the demand estimate. This helps predict in which way and how much each available resource will be needed/used.
How Do You Calculate Forecast Accuracy?

You can use a forecast formula to calculate the accuracy of a particular forecast. The most commonly used forecasting formulas are:
Mean Absolute Percentage Error
The Mean Absolute Percentage Error (MAPE) is a formula used to determine the accuracy of different types of estimates (e.g., time estimates, project budget estimates, sale estimates, etc). The way to calculate MAPE is by subtracting the estimated value (forecast) from the actual value, dividing the result by the actual value, and then multiplying that result by 100 to turn it into percentages.
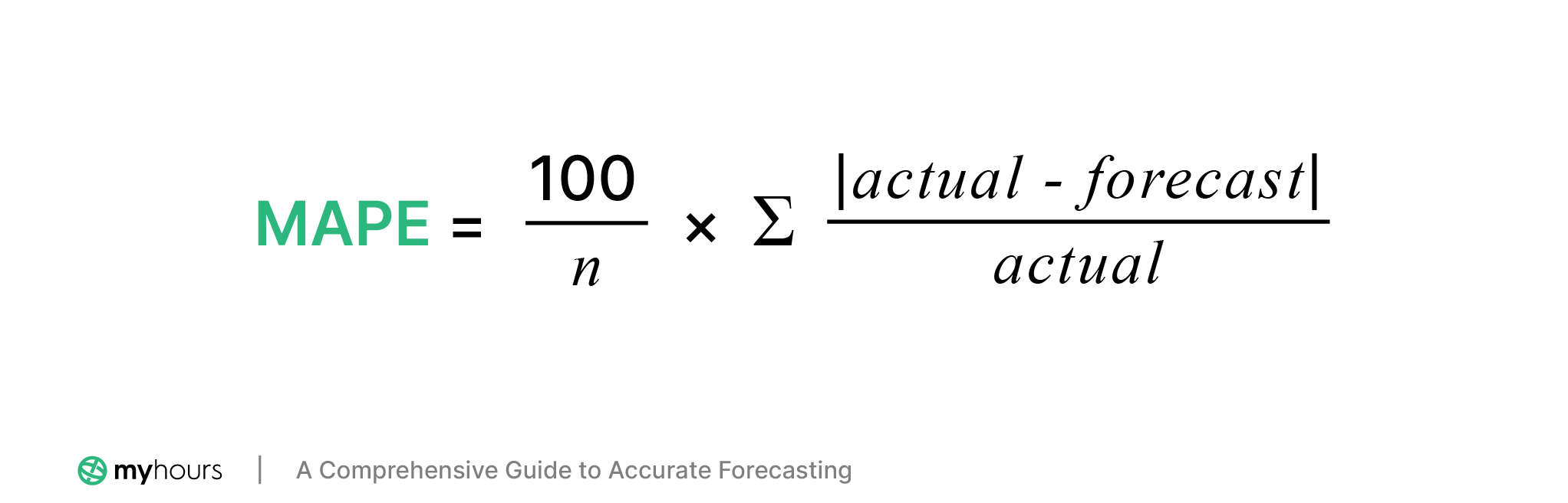
Here’s a short example of MAPE calculation, just to make sure you fully understand how to use this formula:
MAPE Calculation Example
Let’s say you’ve completed a time estimate for a project. The forecast shows that to finish said project your company will need to spend 1250 work hours. You start working on the project, ultimately finishing it in 1500 work hours. Now that you have both the forecast and the actual value (which you got after completing the project), you can determine the forecast accuracy of the particular estimating method you’ve used. Let’s take those numbers and put them into the MAPE formula:
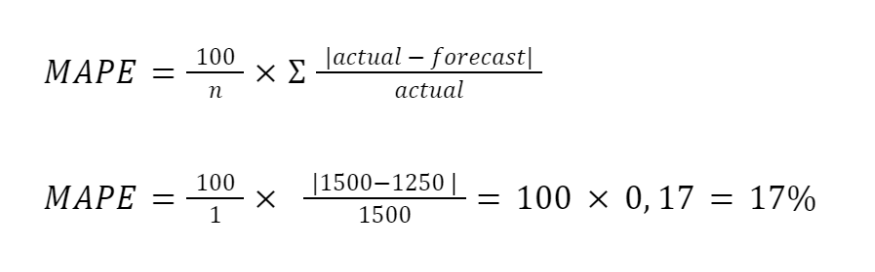
So, in this particular case, the forecast accuracy was off by 17%
Before moving on to the next forecast accuracy formula, we must mention that the MAPE calculation is not always useful. For example, if you’re dealing with small numbers or quantities, you might get results that don’t show the full picture.
Let’s say your forecast says that you’ll need 2 of something (it could be anything, but let’s say work hours) to finish a project. You start working on it and end up completing it in 1 hour. With MAPE, you’ll get a result that shows:
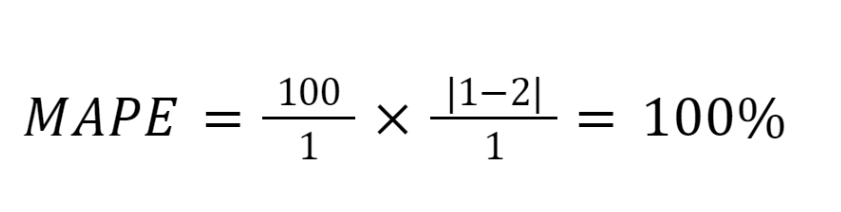
In this case, the forecast was off by 100% (instead of 2, the project took 1 hour to finish). Although technically correct, an error of 100% does not fully reflect the forecast accuracy. The result of 100% suggests that the original estimate was completely off when, in reality, it was only off by a single hour.
Mean Absolute Error
The Mean Absolute Error (MAE) formula for calculating forecast accuracy works by taking the absolute value (i.e., the non-negative value of the number) of the Forecast subtracted by the Actual value, and then dividing that result by the sample size:

MAE Calculation Example
Let’s assume your forecasting method predicts daily prices for a specific item and the price changes daily. Let’s also say that those daily forecasts for that particular item’s price are:
Monday = $10
Tuesday = $12
Wednesday = $9
Thursday = 11$
Friday = $10
Saturday = $12
Sunday = $9
Now, let’s imagine a week has passed, and you got the actual daily value for said item:
Monday = $11
Tuesday = $10
Wednesday = $10
Thursday = 10$
Friday = $9
Saturday = $12
Sunday = $10
Our sample size is 7 (because it considers every day of the week). With that and the numbers from above in mind, the MAE calculation in this case will look something like this:
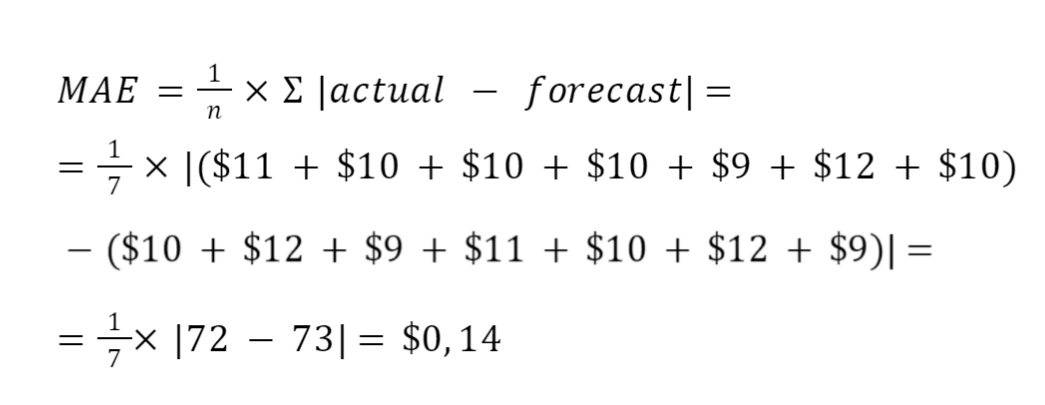
In this example, the MAE calculation indicates that the average prediction error will be $0,14 per sample or data point.
Root Mean Squared Error
The Root Mean Square Error (RMSE) is calculated equal to the square root of the Mean Square Error (MSE). And, MSE is calculated by squaring the difference between the actual and predicted error.
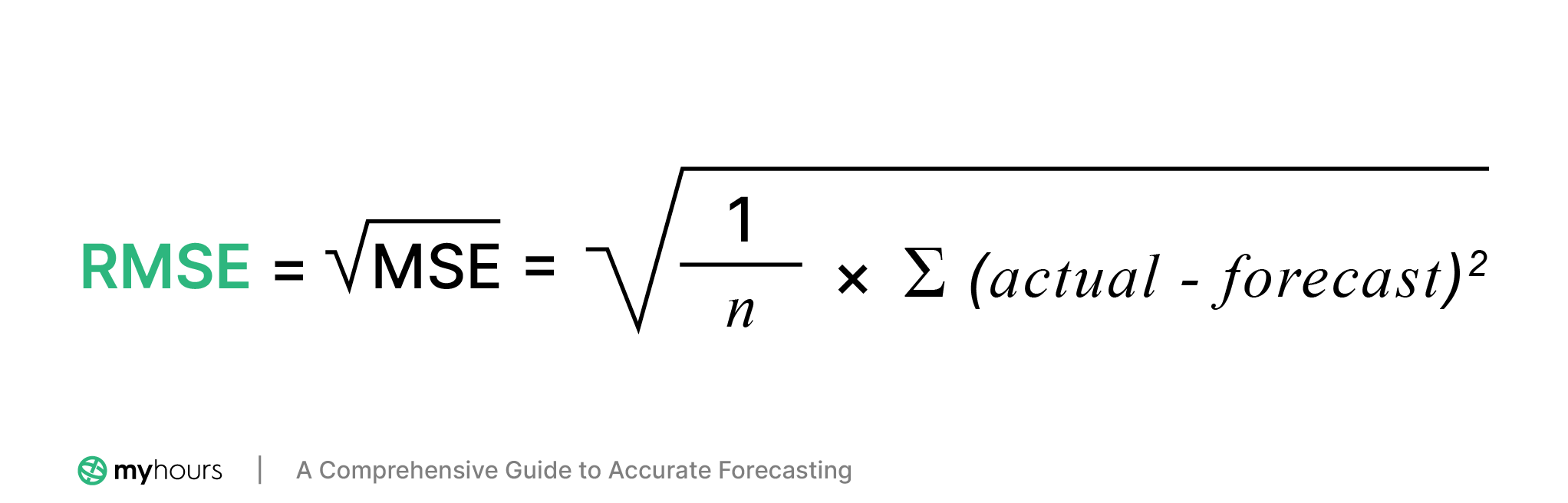
To make this forecast accuracy formula easier to understand, we’ve prepared a short example, which you can find below.
Root Mean Squared Error Calculation Example
Let’s say you’re using a single forecasting model to predict how long four tasks will take. The predicted results you’ve got are:
Task 1 = 10 hours
Task 2 = 12 hours
Task 3 = 14 hours
Task 4 = 8 hours
Now, let’s also assume you’ve used time-tracking software to track how long those tasks actually took. And the results you've got are:
Task 1 = 11 hours
Task 2 = 12 hours
Task 3 = 16 hours
Task 4 = 5 hours
Before calculating the RMSE, we first need to get the MSE. To do that, we should calculate the differences between predicted and actual values for each task and square the difference:

Now that we have our MSE, we can calculate the RMSE by determining the square root of the MSE:
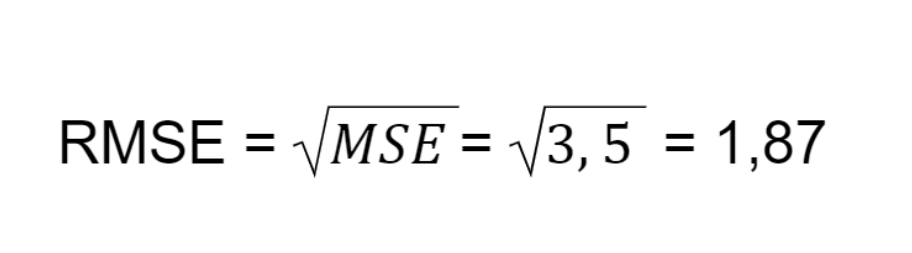
For this example, the Root Mean Square Error is 1,87 hours per data point or sample size.
Common Forecast Accuracy Mistakes and Challenges
There are many challenges and easily avoidable mistakes that can negatively affect forecast accuracy. Some of the most common ones are:
Availability and Accuracy of Data
It's virtually impossible to create precise forecasts without data and, most importantly, accurate data. The more complicated the forecast, the more data it will require from different sources. All of it needs to be correct, stored properly, and easily accessible. If just one of these three things is not up to par, the forecast accuracy will be compromised, and it will also take more time to complete.
Let’s say you’re making a time estimate and need information about task duration. To get it, you need information about how long each employee took to finish a particular task. Suppose that data is not located in a centralized location (e.g., a cloud-based system) from which you can access and gather every data point you need for the forecast. In that case, you’ll need to check task duration with each employee individually. And doing that will take up a lot of time. Not to mention that because the information about tasks will be coming from a human source, which is, as we know, error-prone, the accuracy of the data you collect this way will be compromised.
The best way to avoid availability issues and ensure the data you’re using for the forecast is 100% accurate is to use a cloud-based solution that is easily accessible and contains all the necessary information. And, in the case of time estimates, what you would want to do is use any of the time-tracking apps that allow you to track task duration.
Solely Relying on Work Experience and Gut Feelings
It’s entirely natural for people with a lot of work experience to occasionally rely on their gut feelings when making a forecast. Doing that can definitely pay off, sometimes. But accurate forecasting is not about “sometimes.” It’s about creating accurate prediction models that are right most or all the time. And getting to that point, solely relying on experience or gut feeling, is not possible.
We’re not saying never to trust your gut or work experience when making forecasts. What you should do instead is combine them with relevant data points to make an estimate that is both data- and experience-driven.
Ignoring or Not Having Historical Data
Historical data can be beneficial when making almost any type of forecast. And the more of it you have, the higher the likelihood that your estimate will be accurate. If you’re in sales, historical data can tell you which team members had the best results over a specific period. Historical data can show you which of the products or services you’re selling is the most popular with your target audience, when and how you're generating leads, and what the quality of those leads is. It is something that should never be ignored when making a forecast.
But what if you don’t have any historical data? What if your company doesn’t have systems in place to record relevant values and data points that can be used to create an estimate? The answer is simple, start gathering data now! It doesn't matter in which industry or in what type of business you are, without data, you won’t be able to compete in the modern market.
How to Create Accurate Forecasts: Main Things to Consider
1. Evaluate Stock Levels or Manpower
Make sure you closely evaluate your stock levels, especially the demand and the number of actual sales. If you’re selling services, treat stock levels as your available manpower and the total number of available work hours.
The demand is important because it shows the market need for your products or services. And sales are important because they tell you exactly how much of that market demand turns into sales.
If you have historical data, use it to determine peak and slow periods during the year and analyze what your stock levels were during both. Try to answer questions like:
- Did we miss any sales during the peak period because we were understocked?
- Was the stock level too high during the slow period, and did that increase expenses?
Another thing that you should consider doing is market polls. They are a great way to get instant feedback from customers about your products or services and to learn more about demand for both. It’s also a good idea to consider your competitors and see how their business affects the overall demand and your sales.
2. Take Into Account Payment Delays
It’s not uncommon, especially for B2B companies, to wait a couple of weeks or, in some cases, even months to receive a payment from the sale they’ve made.
Maybe you’re selling products through Amazon. In that case, instead of receiving money immediately after the product is sold, you’ll get it on the next scheduled settlement date (as per your agreement with the company). This means that you can sell a bunch of products but not get payment for them in months. And that can affect your sales numbers. So, before making a forecast, ensure you calculate payment methods into your projection model.
Record any payment delays, and use that information to make your forecast more accurate.
3. Research Your Target Audience
Researching your customer base is one of the most important parts of creating an accurate forecast. The main things you should try to learn about your target audience are the following:
- Demographics — Include factors such as age, gender, education, average pay, and other demographic elements that you feel are important. The more relevant data points you collect, the more accurately you can forecast purchasing behaviors and preferences.
- Buying trends — It’s important to analyze past buying behaviors to accurately predict future buying trends. Evaluate customer information such as purchase history and frequency, the type of products or services they are most likely to pay for, and the reaction of your customer base to your marketing campaigns and strategy (how does that affect sales?).
- Geographic area—Knowing the region of the country or the world where your customer base is located can tell you a lot about the demand and current market trends for that specific geographic location. This can allow you to create more targeted marketing campaigns for specific regions.
- Customer satisfaction and loyalty — Try organizing customer polls to evaluate customer satisfaction and loyalty levels. More satisfied customers are more likely to make repeat purchases of your products or services and can serve as free brand ambassadors and end up promoting your business.
- Seasonal and other trends — Make sure to take into account seasonal or other trends that are impacting your sales and customer behavior. If, for example, a certain group of your customer base only makes purchases during holidays, they are more than likely gift shoppers. To accommodate for that, you can shift your stock levels and sales processes to lower costs during those periods. Make sure to remember to account for those changes when making a forecast.
4. Know Your Competition
To create an accurate forecast, you must learn all you can about your competition. Try to calculate your market share and compare it to your direct competitors. You can use the results to predict your sales volumes somewhat accurately.
For example, let’s say you have 20% of a market that generates around $200,000 per year. In that case, you can roughly estimate your sales at around $40,000 annually.
4 Tips to Increase Forecast Accuracy
1. Use Digital Solutions
Different digital solutions or specialized software can allow you to accurately track specific data points you need for your forecasting model.
Let’s say you’re making a project time estimate because you want to improve project management in your company. One of the data points you’ll need to create your forecast is task duration (i.e., how long did each task of a larger project take to complete). This means that you need a way to track and record the duration of tasks.
Now, you can do this manually. But be aware that the data you get manually might not always be accurate, and if it’s off by a little, it can affect the overall forecast accuracy. Ideally, you would want to use time-tracking software, which automatically tracks employee work hours and the duration of individual tasks.
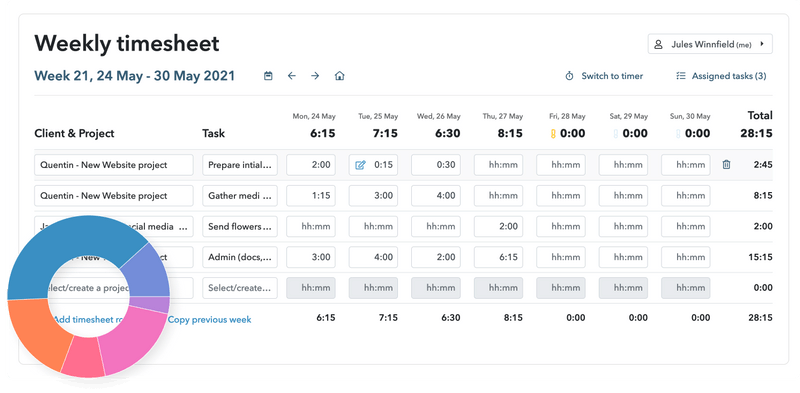
So, no matter what kind of data points you need for your forecasting model, just know that you won't get accurate results if you don’t feed it with accurate information. And one of the best ways to ensure the information you’re using is 100% accurate is to use modern digital solutions.
2. Always Review Your Forecasts
It’s not enough to just create a forecast. For it to work fully and as accurately as possible, you have to review it regularly (e.g., once a month or a quarter, depending on how urgent the forecast is). Examine the results and input new and fresh data points as they become available.
For example, if, during certain months, there’s a spike in interest for your products or services, be sure to add that information to your estimate. Continually adjusting your estimate can ensure their accuracy stays high throughout the year and can help you make adjustments and better evaluate business plans.
3. Consider as Many Data Points as You Can
When it comes to forecasting, using more data points usually translates into a higher forecast accuracy. But, we should also mention that there is a tipping point where too much data will just end up cluttering your projections. So, what do you do?
Let’s say you are 100% sure you need five data points for a particular forecast. But you also see ten other data points that may or may not be relevant to your forecast. The solution here is to add them individually to your forecast and see what comes of it. If they increase the forecast accuracy, keep them. If they don’t, discard them and move on. The main point is that you need to track and record more data points than you’ll use in your forecast.
Be sure to consider as many data points as you deem relevant, track and record them, add them to your forecast, and evaluate how they impact the forecast accuracy.
4. Try out and Combine Different Forecasting Methods
Every forecasting method has certain limitations that can negatively impact forecast accuracy. By trying out different methods, comparing how they fare against each other, and combining them, you can offset the limitations intrinsic to each method.
When you use multiple forecasts to get more accurate results, that’s called ensemble forecasting. It’s sort of like diversity is our strength, but for forecasting. And just like people, diversity makes forecasts more reliable and accurate.
FAQ
How do you set forecast accuracy targets?
To set forecast accuracy targets, you should always:
- Make sure they align with your business goals (sales targets, inventory management goals, or other business objectives)
- Analyze previous forecasts and review their accuracy (if you have used forecasts before)
- Take into account the time period of your forecast (is it a short, medium, or long-term forecast? Short-term forecasts usually have higher forecast accuracy targets as they tend to have an immediate impact on the company’s operation)
- Always involve stakeholders and experts (they can add valuable information about the specific data points you’re using or want to use)
What are the factors affecting forecast accuracy?
Forecast accuracy can be affected by various factors including:
- The quality and quantity of data
- Limitations of a specific forecasting method
- Economic downturns
- Market trends
- Forecasting period
- Seasonal changes
What is a good accuracy forecast percentage?
Most experts would agree that a good accuracy forecast percentage is any percentage above 70%.
It’s important to note that this greatly depends on industry and demand. For example, in retail, where there is a lot of competition and the market is constantly changing, the margin error can be made lower. This is especially true when dealing with flagship products, where most companies won’t accept an accuracy forecast percentage lower than 80%. In this case, a good accuracy forecast percentage would be anything between 80% and 95% (with 100% accuracy being almost unattainable).